REAL-TIME IDENTIFICATION OF MAIZE PLANT AND WEEDS USING MACHINE LEARNING MODEL IMPLEMENTED VIA YOLO v5
DOI:
https://doi.org/10.62050/fjst2024.v8n2.346Keywords:
Agriculture, Machine learning, Maize, Weed, YOLO v5Abstract
Agriculture is as old as the existence of humanity. Early humans engaged in fruit gathering and hunting for food. Agriculture has improved over the years and the need for effective agricultural practices has become a growing concern for farmers. Maize is a staple food in many countries and serves as a primary source of carbohydrates. It is versatile and used for human consumption, animal feed, and industrial applications. Weeds are one of the major obstacles faced in crop production. Weeds compete with plants for nutrients, space and sunlight, affecting the quality of crop yield. This paper aims to use machine learning to train a model to identify weeds and maize plants on the farmland. This research focuses on implementing a real-time detection system using the YOLOv5 (You Only Look Once version 5) deep learning model. The Machine learning model was developed using a training set of about 3000 images of maize plants obtained from the farmland. The obtained data were annotated via makesense.ai and the labelled dataset was divided into training and testing sets. An overall accuracy of over 90 per cent was achieved with the implemented model and a mAP value of 0.95, demonstrating the efficacy of our approach for identifying and differentiating a maize plant from the weeds.
Downloads
References
Abouziena, H. F., & Haggag, W. M. (2016). Weed control in clean agriculture: a review1. Planta daninha, 34(2), 377-392.
Dasgupta, I., Saha, J., Venkatasubbu, P., & Ramasubramanian, P. (2020). AI crop predictor and weed detector using wireless technologies: a smart application for farmers. Arabian Journal for Science and Engineering, 45, 11115-11127.
Du Plessis, J. (2003). Maize production (pp. 1-38). Pretoria, South Africa: Department of Agriculture.
Eckhoff, S. R., & Paulsen, M. R. (1996). Maize. In Cereal grain quality (pp. 77-112). Dordrecht: Springer Netherlands.
Knezevic, S. Z., Pavlovic, P., Osipitan, O. A., Barnes, E. R., Beiermann, C., Oliveira, M. C., & Jhala, A. (2019). Critical time for weed removal in glyphosate-resistant soybean as influenced by preemergence herbicides. Weed Technology, 33(3), 393-399.
Liebman, M., C. L. Mohler, and C. P. Staver. 2001. Ecological management of agricultural weeds. Cambridge University Press, New York.
Liu, B., Bruch, R. Weed Detection for Selective Spraying: a Review. Curr Robot Rep 1, 19–26 (2020). https://doi.org/10.1007/s43154-020-00001-w
Mazzia. V, A. Khaliq, F. Salvetti and M. Chiaberge, "Real-Time Apple Detection System Using Embedded Systems With Hardware Accelerators: An Edge AI Application," in IEEE Access, vol. 8, pp. 9102-9114, 2020, doi: 10.1109/ACCESS.2020.2964608
Mhlanga, B., Chauhan, B. S., & Thierfelder, C. (2016). Weed management in maize using crop competition: A review. Crop Protection, 88, 28-36.
Otorkpa, O. J. (2017). Health impact of the indiscriminate use of herbicides in Nigeria. Texila International Journal of Public Health, 5(1), 1-9.
Pontes Junior, V.B., Alberto da Silva, A., D'Antonino, L., Mendes, K.F., de Paula Medeiros, B.A. (2022). Methods of Control and Integrated Management of Weeds in Agriculture. In: Mendes,
Rajcan, I., & Swanton, C. J. (2001). Understanding maize–weed competition: resource competition, light quality and the whole plant. Field crops research, 71(2), 139-150.
Shrinivas, C. S. (2016). A review on weed management on maize (Zea mays L.). Advances in Life Sciences, 5(9), 3448-3455.
Sharma, N., & Rayamajhi, M. (2022). Different aspects of weed management in maize (Zea mays L.): A brief review. Advances in Agriculture, 2022(1), 7960175.
Venkataraju, A., Arumugam, D., Stepan, C., Kiran, R., & Peters, T. (2023). A review of machine learning techniques for identifying weeds in corn. Smart Agricultural Technology, 3, 100102.
Wang, N., Zhang, N., Wei, J., Stoll, Q., & Peterson, D. E. (2007). A real-time, embedded, weed-detection system for use in wheat fields. Biosystems Engineering, 98(3), 276-285.
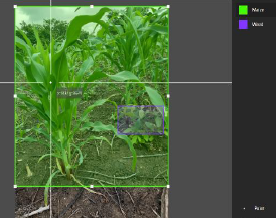